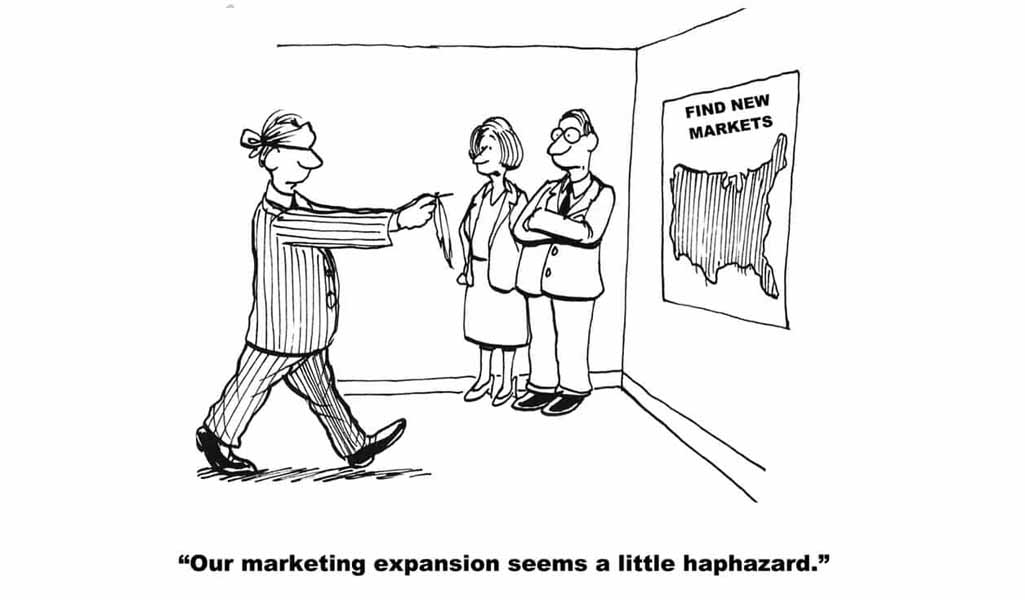
“Precision Segmentation” – Finding Buyers in the Digital Age
“Oh my,” you say, “not another blog about segmentation, something I know a lot about. I learned about market segmentation back in my Marketing 101 class.” Well, digital marketing and machine learning leave much of that old class material in need of severe upgrading and updating. So, don’t click that back button quite yet and read on, instead.
We can now define segments much more precisely using dozens or even hundreds of dimensions, not just the usual standby segmentation filters of vertical, geography, size, or titles, i.e., a much more precise and thus more success-prone definition of to-be-targeted segments can be obtained. And we can measure the degree of our success hitting the right targets in near real-time, i.e., getting market feedback to our targeting way more quickly. This means that “getting it right the first time” is not as necessary, and it’s more important to be able to rapidly collect lots of data on the rise of demand, process it, and adjust course if and as needed.
Why do precision segmentation at all?
Add to the top line: Ultimately, the reason to identify resonating market segments is that lead volumes, conversion and closure rates, sales velocities, and/or average deal sizes will go up, in some cases dramatically, as we showed in Figure 1. In contrast to the MBA reasoning of old, nowadays, we can measure changes to those parameters after a few weeks or months into a new go-to-market launch. And the faster we can measure them, the sooner we’ll hit those promising market segments with high resonance.
For example, underlying resonances can be established more readily: Increases in open or click-through rates in search engine ads or email campaigns into targeted segments give near-immediate feedback about the interest of a targeted sector in your offerings. In the olden days, it required live customer interviews and detailed sales analyses that maybe were available after a few months. Or decision-makers had to rely on impressionistic data and intuition. Now you’ll know days or weeks at most into a campaign.
Add to your bottom-line: Not only are there the above improvements to a company’s top-line, but the bottom-line also improves as well through the more efficient purchase of databases and online advertising. You only need to buy the leads and ads that precisely speak to those you are targeting. For many B2B businesses, the to-be-targeted population often contains only a few hundred or maybe a couple of thousands of decision-makers. What if you only had to know who they are and create ways only to reach them? Much cheaper than Super Bowl ads or blanket database purchases that telesales reps then cold call into with low closure rates.
A lot is also about messaging to allow organic traffic to your web assets to filter themselves in or out. Which means, if they’re not interested, they won’t even show up on your radar screen, and you don’t have to waste time with them.

Figure 1: Differential Closure Rates by Segment
What is different about precision segmentation in digital marketing?
So, say hi to precision segmentation in digital marketing along deep firmographic, demographic, and psychographic dimensions using machine learning techniques and in-depth analysis of CRM data, as well as a wealth of online, behavioral data. Much of that didn’t exist a few years ago, and so much of the needed know-how is still tribal knowledge shared among innovative practitioners. So, here is a summary of our practitioner experience in segmenting markets in the new age with a focus on leveraging these new approaches to create more high-quality pipeline and lower the costs of doing so.
The significant difference between segmentation in the digital age and more conventional “MBA school-type, segmentation analyses” is not that segmentation isn’t about product-market fit and finding the segments that resonate with your value proposition anymore; it still is. The difference is in the techniques and the speed with which a more significant number of circumscribing filters that define resonant segments can be established, and therefore the degree of targeting precision that is possible now.
A few illustrative examples of how segmentation can be more precise:
Accurate CRM Data:
Resonating segments might still be verticals with specific needs that match a company’s product strengths. One eHealth company discovered through a detailed analysis of their CRM system’s data (i.e., lead volumes, conversion rates from one first stage to the next, and sales velocities for different segments) that their application was tailor-made for the regulatory needs of the medical device industry and thus saw significantly increased closure rates there (see Figure 1). Two such high-resonance segments had around 4X the closure rates than the low resonance segments, all other things being equal. Such insights, impossible to obtain without detailed CRM data, can have profound implications for how much revenue a given sales and marketing team can generate.
Non-traditional Segmentation Like Social Proximity:
Other examples are from several professional services businesses that we served whose original targeting was along conventional, vertical lines. Only to discover that the segments where they had resonance weren’t associated with specific verticals at all, but with a mixture of company size (medium to lower enterprise, in most cases) and, more importantly, “social proximity.” i.e., they mostly succeeded in selling to folks that either knew them already or that had been referred to them by someone that knew or had worked with them. That’s a common theme in services: Whether it’s your doctor, car mechanic, creative agency, or a consultancy, for services, we tend to go to the folks we trust. And trust mostly comes from close social proximity. So, what worked for them was figuring out how to target new prospects in their respective networks or at most one connection away. With tools like LinkedIn, that kind of targeting is quickly done now.
Segment Filters Obtainable Through Deep Web Searches:
A third example was a company with an online trade credit application that realized that segmentation along vertical lines was only partially significant. Other, less readily discernable factors determined their areas of resonance like the age of their target companies, their ability to secure lines of credit or not, and their need to compete with larger, better-funded companies. Less tangible filters like the ones just cited could not be obtained with traditional segmentation analyses. Still, they can be obtained through modern, machine learning-based techniques that can flush out filters with high closure rate correlation from scraped data off the internet or from in-depth analyses of CRM data looking first volumes, conversion rates, and sales velocities by segment.
Launching a Brand-New Segment or Technology:
How can we segment those markets? For startups that are launching disruptive products, the ability to leverage the before-mentioned increases in speed and precision by understanding new techniques and technologies is significant. Extrapolating from past data isn’t all that relevant for disruptive products, and the speed with which revenue ramps need to be scaled doesn’t allow for months-long strategic research projects anymore. Market segments and sub-segments need to be identified, tested, and expanded or discarded in a matter of a few weeks or months these days.
Where to start – how to find your high-resonance segments?
At the end, where segmentation definitions manifest themselves are in concrete decisions around the design and number of attributes of the lead databases that get purchased or enriched, the selection of targeting filters in advertising platforms like LinkedIn, Facebook or Google, as well as in the associated messaging and underlying value propositions. The latter allowing readers to self-select if they want to become prospects because they resonate with what is being said, or if they’re going to self-select out.
Setting up the data collection mechanisms to what segment attributes to filter in or filter out is vital, and at least in the beginning can be done easily. A/B testing Google or LinkedIn ads, looking at email open and click-through rates, monitoring social media engagement rates are not difficult, yet if collected regularly and mapped back against the tested variables will provide insights very quickly.
So, segmentation these days is more about what databases to buy, who to advertise to with what messaging, and about who to contact on LinkedIn or Facebook. And if we don’t get it right in the first throw, it’s good enough to get close and iterate into a resonating target segment(s) through data-driven iterations. The market might surprise us with who is interested if we just expose ourselves to them versus over-defining a target segment and exclusively focusing on it, missing out on attractive others.
So, rather than defining what the segmentation should be and then running demand generation against that definition, we now can instead define a few segments, run some cheap and early campaigns, and measure their differential uptake. Then refine quickly over the ensuing 3 to 12 months and step on the gas in the (sub)segments where market resonance has been established.
Here is a straight-forward process to identify high resonance segments:
- Analyze the last 5 or 10 wins and losses (or early prospect discussions if your organization is too young to have sales yet), writing down answers to these questions:
- How did you find them/they find you?
- In their own words (not yours), why are they interested / did they buy / not buy?
- What did they / did they not like?
- Capture any segmentation information you might be able to ascertain like company size, geo, vertical, profitability, etc. about the company, and things like title, gender, attitudes about innovation, personal motivations, social proximity, etc. about the buyer(s)
- Can you detect any apparent correlations that might give you a sense of what their defining commonalities are? Sometimes segment commonalities / shared filtering attributes take a while to surface and often can be counter-intuitive.
- Armed with that information, then run regression analyses (Excel has capabilities that are good enough with their “LINEST” function) against CRM data using the above criteria as a source list of filters to start. This will allows you to see which of the measurable portions of the above filters correlate with sales volume, conversion and closure rates, sales velocities.
- Once segmentation filters/dimensions/attributes have been identified in steps 1 and 2, then the task becomes where and how we can obtain leads that match these segmentation criteria. Some ways to do so include:
- First comb through commercially available databases that offer leads enriched with the types of segment attributes you are looking for (e.g., gender, age, educational attainment, other vendors deployed at a prospect site, etc.) and verify they are of high enough quality
- Second, using vendors skilled in using machine-learning techniques to do deep web searches and separate the attributes of your buyers and prospects from everyone else.
- Validate the findings with a few live interviews to make sure they pass a qualitative acid test
- Translate into:
- Clear definition of prospect/customer journeys and where and how to intercept them
- Precise definitions of database purchases
- Launch of highly targeted ads (Google, LinkedIn, FB, ad retargeting, communities)
- Online and collateral messaging.
When executed, there is better product-market fit, higher conversion, and closure rates, and less wasted marketing dollars i.e., more revenues and lower costs.